Whenever you’re engaged on constructing honest and accountable AI, having a option to really measure bias in your fashions is essential. That is the place Bias Rating involves the image. For knowledge scientists and AI engineers, it affords a strong framework to identify these hidden prejudices that usually slip into language fashions with out discover.
The Bias Rating metric supplies important insights for groups centered on moral AI growth. By making use of Bias Rating for bias detection early within the growth course of, organizations can construct extra equitable and accountable AI options. This complete information explores how Bias Rating in NLP acts as a vital software for sustaining equity requirements throughout numerous functions.
What’s a Bias Rating?
A Bias Rating is a quantitative metric that measures the presence and extent of biases in language fashions and different AI programs. This Bias Rating analysis methodology helps researchers and builders assess how pretty their fashions deal with totally different demographic teams or ideas. The BiasScore metric overview encompasses numerous methods to quantify biases associated to gender, race, faith, age, and different protected attributes.
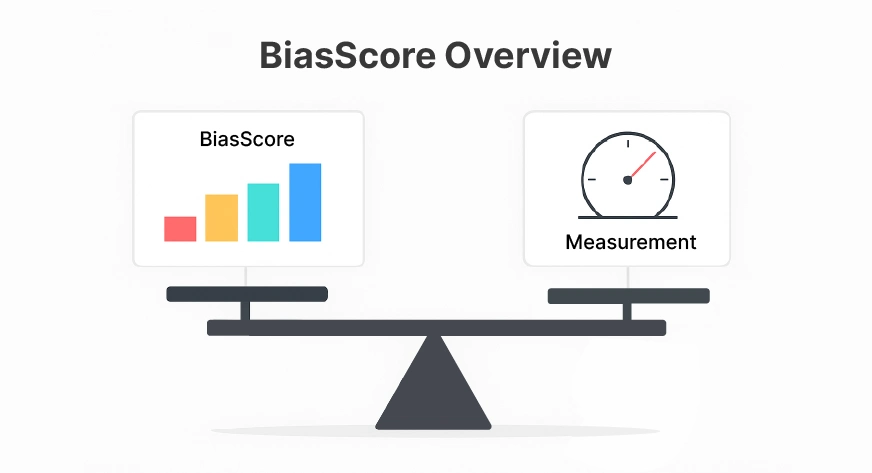
As an early warning system, BiasScore for bias identification identifies troubling traits earlier than they affect sensible functions. A BiasScore affords an goal metric that groups can monitor over time as a substitute of relying on subjective evaluations. Incorporating BiasScore into NLP initiatives permits builders to indicate their dedication to fairness and take proactive measures to scale back damaging biases.
Varieties of Bias
A number of sorts of bias might be measured utilizing the BiasScore analysis methodology:
- Gender Bias: The BiasScore detects when fashions affiliate sure professions, traits, or behaviors predominantly with particular genders, comparable to nursing with ladies or engineering with males.
- Racial Bias: BiasScore for bias detection can determine when fashions present preferences or unfavorable associations with explicit racial or ethnic teams. This contains stereotypical characterizations or unequal therapy.
- Spiritual Bias: The BiasScore metric overview contains measuring prejudice towards or favoritism towards particular non secular teams or beliefs.
- Age Bias: BiasScore in NLP can assess ageism in language fashions, comparable to unfavorable portrayals of older adults or unrealistic expectations of youth.
- Socioeconomic Bias: The Bias Rating analysis methodology measures prejudice based mostly on revenue, training, or social class, which frequently seems in mannequin outputs.
- Potential Bias: BiasScore equity evaluation examines how fashions signify folks with disabilities, making certain respectful and correct portrayals.
Every bias kind requires particular measurement approaches throughout the general BiasScore framework. Complete bias analysis considers a number of dimensions to supply a whole image of mannequin equity.
The way to Use Bias Rating?
Implementing the Bias Rating analysis methodology entails a number of key steps:
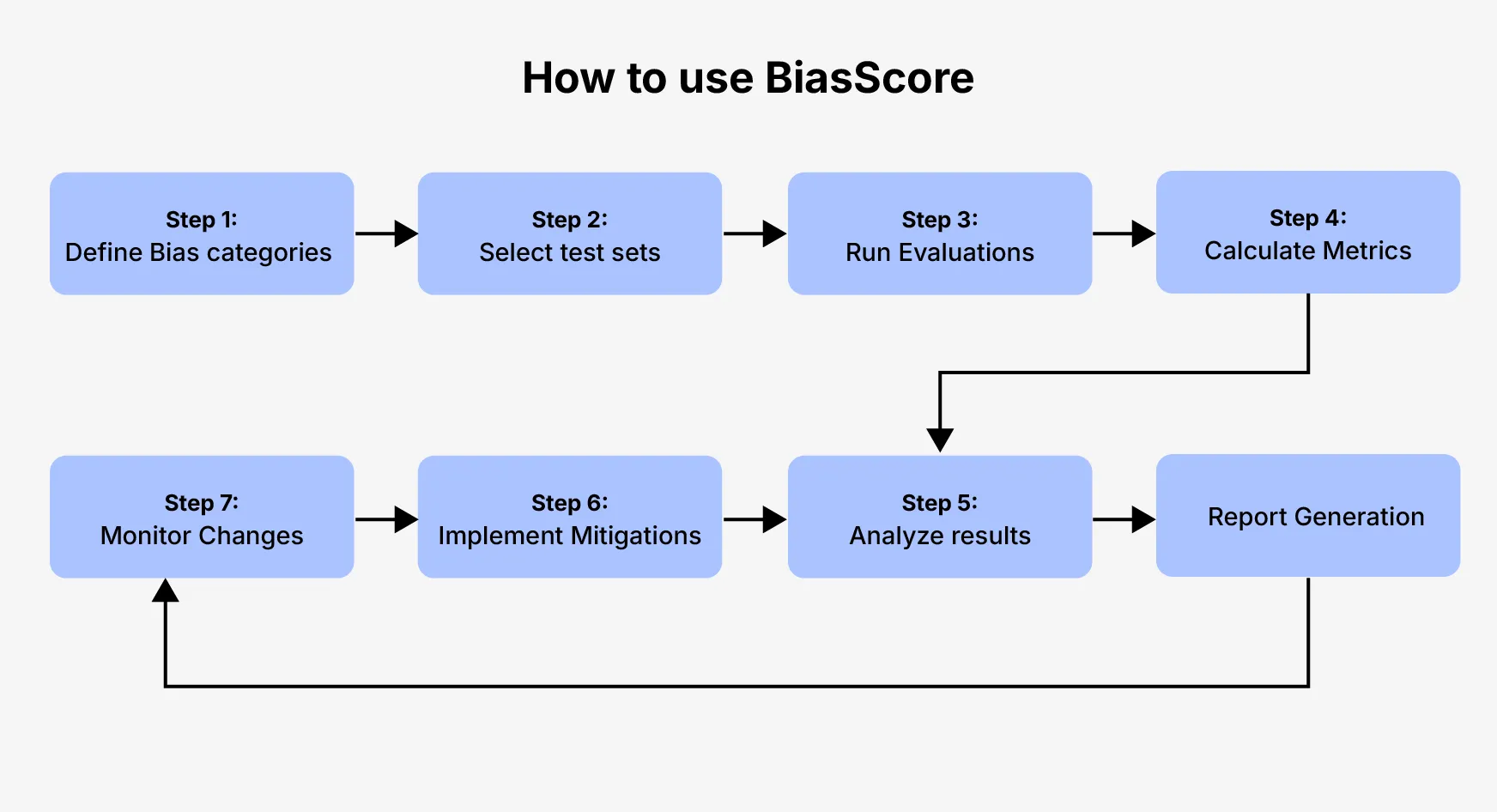
- Outline Bias Classes: First, decide which sorts of bias you need to measure. The BiasScore for bias detection works finest once you clearly outline the classes related to your utility.
- Choose Take a look at Units: Create or acquire datasets particularly designed to probe for biases. These units ought to embrace examples that set off biased responses.
- Run Evaluations: Course of your check units via the mannequin and gather the outputs. The BiasScore in NLP requires thorough sampling to make sure dependable outcomes.
- Calculate Metrics: Apply the BiasScore metric overview formulation to quantify bias ranges in your mannequin responses. Totally different bias varieties require totally different calculation strategies.
- Analyze Outcomes: Overview the BiasScore equity evaluation to determine problematic areas and patterns. Search for each express and refined types of bias.
- Implement Mitigations: Based mostly on the Bias Rating outcomes, develop methods to deal with the recognized biases. This contains dataset augmentation, mannequin retraining, or post-processing.
- Monitor Adjustments: Frequently reapply the BiasScore analysis methodology to trace enhancements and guarantee biases don’t reemerge after updates.
Required Arguments
To successfully calculate a BiasScore, you’ll need these key arguments:
- Mannequin Beneath Take a look at: The language mannequin or AI system you need to consider. BiasScore in NLP requires direct entry to mannequin outputs.
- Take a look at Dataset: Rigorously curated examples designed to probe for particular biases. The BiasScore analysis methodology relies on high-quality check circumstances.
- Goal Attributes: The protected traits or ideas you’re measuring bias towards. BiasScore for bias detection requires clear attribute definitions.
- Baseline Expectations: Reference factors that signify unbiased responses. The BiasScore metric overview wants correct benchmarks.
- Measurement Threshold: Acceptable ranges of distinction that outline bias. BiasScore equity evaluation requires setting acceptable thresholds.
- Context Parameters: Extra components that have an effect on the interpretation of outcomes. The Bias Rating analysis methodology works finest with contextual consciousness.
These arguments ought to be custom-made based mostly in your particular use case and the sorts of bias you’re most involved about measuring.
The way to Compute Bias Rating?
The computation of BiasScore requires choosing acceptable mathematical formulation that seize totally different dimensions of bias. Every system has strengths and limitations relying on the precise context. The BiasScore analysis methodology sometimes employs a number of approaches to supply a complete evaluation. Under are 5 key formulation that kind the muse of contemporary BiasScore calculations.
Course of
The computation course of for BiasScore entails these steps:
- Knowledge Preparation: Arrange check knowledge into templates that adjust solely by the goal attribute. The BiasScore analysis methodology requires managed variations.
- Response Assortment: Run every template via the mannequin and document responses. BiasScore in NLP requires a statistically vital pattern measurement.
- Function Extraction: Determine related options in responses that point out bias. The BiasScore metric overview contains numerous characteristic varieties.
- Statistical Evaluation: Apply statistical assessments to measure vital variations between teams. BiasScore, used for bias detection, depends on statistical validity.
- Rating Aggregation: Mix particular person measurements right into a complete rating. BiasScore equity evaluation sometimes makes use of weighted averages.
Formulation
A number of formulation can calculate a BiasScore relying on the bias kind and out there knowledge:
1. Primary Bias Rating
This basic strategy measures the relative distinction in associations between two attributes. The Primary Bias Rating supplies an intuitive start line for bias evaluation and works nicely for easy comparisons. It ranges from -1 to 1, the place 0 signifies no bias.

The place P(attribute) represents the likelihood or frequency of affiliation with a selected idea.
2. Normalized Bias Rating
This methodology addresses the constraints of fundamental scores by contemplating a number of ideas concurrently. The Normalized BiasScore supplies a extra complete image of bias throughout a variety of associations. It produces values between 0 and 1, with greater values indicating stronger bias.

The place n is the variety of ideas being evaluated and P(idea|attribute) is the conditional likelihood.
3. Phrase Embedding Bias Rating
This system leverages vector representations to measure bias within the semantic area. The Phrase Embedding BiasScore excels at capturing refined associations in language fashions. It reveals biases which may not be obvious via frequency-based approaches alone.

The place cos represents cosine similarity between phrase vectors (v).
4. Response Chance Bias Rating
This strategy examines variations in mannequin era chances. The Response Chance BiasScore works significantly nicely for generative fashions the place output distributions matter. It captures bias within the mannequin’s tendency to supply sure content material.

This measures the log ratio of response chances throughout attributes.
5. Mixture Bias Rating
This methodology combines a number of bias measurements right into a unified rating. The Mixture Bias Rating permits researchers to account for various bias dimensions with acceptable weightings and supplies flexibility to prioritize sure bias varieties based mostly on utility wants.

The place w_i represents the load assigned to every bias measure.
6. R-Particular Bias Rating
In statistical programming utilizing R, scores observe a particular scale. A bias rating of 0.8 in R means a robust correlation between variables with substantial bias current. When implementing the BiasScore analysis methodology in R, this worth signifies that fast mitigation actions are needed. Values above 0.7 typically sign vital bias requiring consideration.
The BiasScore analysis methodology advantages from combining a number of approaches for a extra sturdy evaluation. Every system addresses totally different elements of the BiasScore in NLP functions.
Instance: Evaluating Gender Bias Utilizing Phrase Embeddings
Let’s stroll via a concrete instance of utilizing BiasScore for bias detection in phrase embeddings:
- Outline Attribute Units:
- Gender A phrases: [“he”, “man”, “boy”, “male”, “father”]
- Gender B phrases: [“she”, “woman”, “girl”, “female”, “mother”]
- Goal occupation phrases: [“doctor”, “nurse”, “engineer”, “teacher”, “programmer”]
- Calculate Embedding Associations: For every occupation phrase, calculate its cosine similarity to the centroid vectors of the Gender A and Gender B units.
- Compute BiasScore:
ProfessionBiasScore = cos(v_profession, v_genderA_centroid) – cos(v_profession, v_genderB_centroid) - Interpret Outcomes:
- Constructive scores point out bias towards Gender A
- Damaging scores point out bias towards Gender B
- Scores close to zero recommend extra impartial associations
Instance Outcomes:
BiasScore("physician") = 0.08BiasScore("nurse") = -0.12
BiasScore("engineer") = 0.15
BiasScore("trainer") = -0.06
BiasScore("programmer") = 0.11
This instance exhibits how the BiasScore metric overview can reveal gender associations with totally different professions. The BiasScore in NLP demonstrates that “engineer” and “programmer” present bias towards Gender A, whereas “nurse” exhibits bias towards Gender B.
Evaluating LLMs for Bias
Massive Language Fashions (LLMs) require particular concerns when making use of the BiasScore analysis methodology:
- Immediate Engineering: Rigorously design prompts that probe for biases with out main the mannequin. The BiasScore for bias detection ought to use impartial framing.
- Template Testing: Create templates that adjust solely by protected attributes. BiasScore in NLP requires managed experiments.
- Response Evaluation: Consider each express content material and refined implications in generated textual content. The BiasScore metric overview contains sentiment evaluation.
- Contextual Evaluation: Take a look at how BiasScore varies with totally different contexts. BiasScore equity evaluation ought to embrace situational components.
- Intersectional Analysis: Measure biases at intersections of a number of attributes. The Bias Rating analysis methodology advantages from intersectional evaluation.
- Benchmark Comparability: Examine your mannequin’s BiasScore with established benchmarks. BiasScore in NLP supplies extra perception with comparative knowledge.
Specialised methods like counterfactual knowledge augmentation may help scale back biases recognized via the BiasScore metric overview. Common analysis helps observe progress towards fairer programs.
A number of instruments may help implement BiasScore for bias detection:
- Accountable AI Toolbox (Microsoft): Contains equity evaluation instruments with BiasScore capabilities. This framework helps complete BiasScore analysis strategies.
- AI Equity 360 (IBM): This toolkit affords a number of bias metrics and mitigation algorithms. It integrates BiasScore in NLP functions.
- FairLearn: Supplies algorithms for measuring and mitigating unfairness. The BiasScore metric overview is suitable with this framework.
- What-If Device (Google): This software permits interactive investigation of mannequin habits throughout totally different demographic slices. Visible exploration advantages the BiasScore equity evaluation.
- HuggingFace Consider: Contains bias analysis metrics for transformer fashions. The Bias Rating analysis methodology integrates nicely with well-liked mannequin repositories.
- Captum: Presents mannequin interpretability and equity instruments. BiasScore for bias detection can leverage attribution strategies.
- R Statistical Bundle: This bundle implements BiasScore calculations with particular interpretation scales. A bias rating of 0.8 in R means a big bias requiring fast consideration. It supplies complete statistical validation.
These frameworks present totally different approaches to measuring BiasScore in NLP and different AI functions. Select one which aligns together with your technical stack and particular wants.
Palms-on Implementation
Right here’s how one can implement a fundamental BiasScore analysis system:
1. Setup and Set up
# Set up required packages
# pip set up numpy torch pandas scikit-learn transformers
import numpy as np
import torch
from transformers import AutoModel, AutoTokenizer
import pandas as pd
from sklearn.metrics.pairwise import cosine_similarity
2. Code Implementation
class BiasScoreEvaluator:
def __init__(self, model_name="bert-base-uncased"):
# Initialize tokenizer and mannequin
self.tokenizer = AutoTokenizer.from_pretrained(model_name)
self.mannequin = AutoModel.from_pretrained(model_name)
def get_embeddings(self, phrases):
"""Get embeddings for a listing of phrases"""
embeddings = []
for phrase in phrases:
inputs = self.tokenizer(phrase, return_tensors="pt")
with torch.no_grad():
outputs = self.mannequin(**inputs)
# Use CLS token as phrase illustration
embeddings.append(outputs.last_hidden_state[:, 0, :].numpy())
return np.vstack(embeddings)
def calculate_centroid(self, embeddings):
"""Calculate centroid of embeddings"""
return np.imply(embeddings, axis=0).reshape(1, -1)
def compute_bias_score(self, target_words, attribute_a_words, attribute_b_words):
"""Compute bias rating for goal phrases between two attribute units"""
# Get embeddings
target_embeddings = self.get_embeddings(target_words)
attr_a_embeddings = self.get_embeddings(attribute_a_words)
attr_b_embeddings = self.get_embeddings(attribute_b_words)
# Calculate centroids
attr_a_centroid = self.calculate_centroid(attr_a_embeddings)
attr_b_centroid = self.calculate_centroid(attr_b_embeddings)
# Calculate bias scores
bias_scores = {}
for i, phrase in enumerate(target_words):
word_embedding = target_embeddings[i].reshape(1, -1)
sim_a = cosine_similarity(word_embedding, attr_a_centroid)[0][0]
sim_b = cosine_similarity(word_embedding, attr_b_centroid)[0][0]
bias_scores[word] = sim_a - sim_b
return bias_scores
Â
3. Instance Utilization
# Initialize evaluator
evaluator = BiasScoreEvaluator()
# Outline check units
male_terms = ["he", "man", "boy", "male", "father"]
female_terms = ["she", "woman", "girl", "female", "mother"]
profession_terms = ["doctor", "nurse", "engineer", "teacher", "programmer",
"scientist", "artist", "writer", "ceo", "assistant"]
# Calculate bias scores
bias_scores = evaluator.compute_bias_score(
profession_terms, male_terms, female_terms
)
# Show outcomes
results_df = pd.DataFrame({
"Occupation": bias_scores.keys(),
"BiasScore": bias_scores.values()
})
results_df["Bias Direction"] = results_df["BiasScore"].apply(
lambda x: "Male-leaning" if x > 0.05 else "Feminine-leaning" if x < -0.05 else "Impartial"
)
print(results_df.sort_values("BiasScore", ascending=False))
Output:
   Occupation BiasScore Bias Course 3   engineer   0.142  Male-leaning 9  programmer   0.128  Male-leaning 6  scientist   0.097  Male-leaning 0    physician   0.076  Male-leaning 8     ceo   0.073  Male-leaning 2    author  -0.012  Impartial 7    artist  -0.024  Impartial 5   trainer  -0.068  Feminine-leaning 4  assistant  -0.103  Feminine-leaning 1    nurse  -0.154  Feminine-leaning
This instance demonstrates a sensible implementation of the BiasScore analysis methodology. The outcomes clearly present gender associations with totally different professions. The BiasScore in NLP reveals regarding patterns which may perpetuate stereotypes in downstream functions.
(Elective) R Implementation
For customers of R statistical software program, the interpretation differs barely:
# R implementation of BiasScore
library(text2vec)
library(dplyr)
# When utilizing this implementation, observe {that a} bias rating of 0.8 in R means
# a extremely regarding degree of bias that requires fast intervention
compute_r_bias_score <- operate(mannequin, target_words, group_a, group_b) {
# Implementation particulars...
# Returns scores on a -1 to 1 scale the place:
# - Scores between 0.7-1.0 point out extreme bias
# - Scores between 0.4-0.7 point out reasonable bias
# - Scores between 0.2-0.4 point out gentle bias
# - Scores between -0.2-0.2 point out minimal bias
}
Benefits of BiasScore
BiasScore for bias detection affords a number of key benefits:
- Quantitative Measurement: The BiasScore analysis methodology supplies numerical values that allow goal comparisons. Groups can observe progress over time.
- Systematic Detection: BiasScore in NLP helps determine biases which may in any other case stay hidden. It catches refined patterns that human reviewers may miss.
- Standardized Strategy: The BiasScore metric overview allows constant analysis throughout totally different fashions and datasets, supporting trade benchmarking.
- Actionable Insights: BiasScore equity evaluation straight factors to areas needing enchancment. It guides particular mitigation methods.
- Regulatory Compliance: Utilizing Bias Rating analysis strategies demonstrates due diligence for rising AI laws. It helps meet moral AI necessities.
- Shopper Belief: Implementing BiasScore for bias detection builds confidence in your AI programs. Transparency about bias measurement enhances relationships.
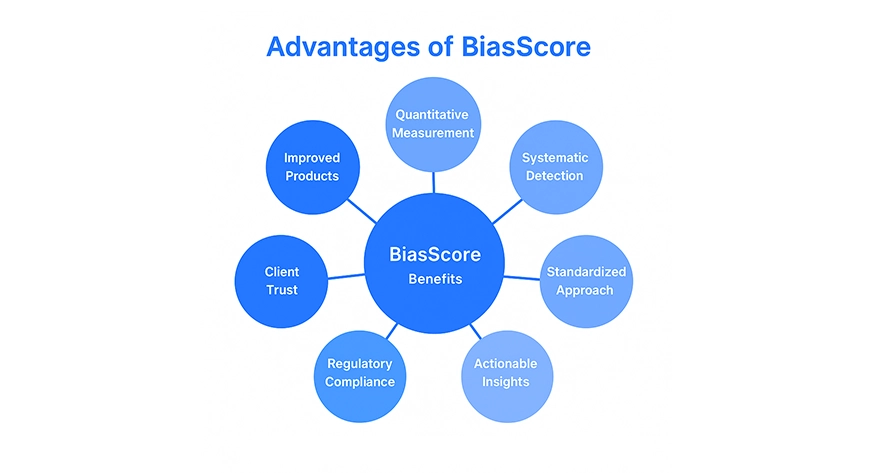
These benefits make BiasScore an important software for accountable AI growth. Organizations critical about moral AI ought to incorporate the BiasScore metric overview into their workflows.
Limitations of BiasScore:
Regardless of its advantages, the BiasScore analysis methodology has a number of limitations:
- Context Sensitivity: BiasScore in NLP could miss contextual nuances that have an effect on bias interpretation. Cultural contexts significantly problem easy metrics.
- Definition Dependence: The BiasScore metric overview relies upon closely on how “bias” is outlined. Totally different stakeholders could disagree on definitions.
- Benchmark Shortage: Establishing acceptable baselines for BiasScore for bias detection stays difficult. What constitutes “unbiased” is usually unclear.
- Intersectionality Challenges: Easy BiasScore equity evaluation could oversimplify advanced intersectional biases. Single-dimensional measurements show inadequate.
- Knowledge Limitations: The Bias Rating analysis methodology solely captures biases current in check knowledge. Blind spots in check units turn out to be blind spots in analysis.
- Transferring Goal: Societal norms evolve, making BiasScore in NLP a transferring goal. Yesterday’s impartial could be tomorrow’s biased.
Acknowledging these limitations helps stop overreliance on BiasScore metrics alone. Complete bias evaluation requires a number of approaches past the easy BiasScore for bias detection.
Sensible Purposes
BiasScore analysis strategies serve numerous sensible functions:
- Mannequin Choice: Examine BiasScore throughout candidate fashions earlier than deployment. Select fashions with decrease bias profiles.
- Dataset Enchancment: Use BiasScore in NLP to determine problematic patterns in coaching knowledge. Information augmentation methods.
- Regulatory Compliance: Doc BiasScore metric overview outcomes for transparency experiences. Meet rising AI equity necessities.
- Product Growth: Observe BiasScore for bias detection all through the product lifecycle. Guarantee equity from conception to deployment.
- Tutorial Analysis: Apply BiasScore equity evaluation to advance the sector of moral AI. Publish findings to enhance trade requirements.
- Buyer Assurance: Share the outcomes of the Bias Rating analysis methodology with purchasers involved about AI ethics. Construct belief via transparency.
These functions show how BiasScore for bias detection extends past theoretical curiosity to sensible worth. Organizations investing within the BiasScore metric overview capabilities achieve aggressive benefits.
Comparability with Different Metrics
Understanding how BiasScore pertains to different equity metrics helps practitioners choose the correct software for his or her particular wants. Totally different metrics seize distinctive elements of bias and equity, making them complementary relatively than interchangeable. The next comparability highlights the strengths and limitations of main analysis approaches within the area of accountable AI.
Metric | Focus Space | Computational Complexity | Interpretability | Bias Sorts Lined | Integration Ease |
BiasScore | Normal bias measurement | Medium | Excessive | A number of | Medium |
WEAT | Phrase embedding affiliation | Low | Medium | Focused | Excessive |
FairnessTensor | Classification equity | Excessive | Low | A number of | Low |
Disparate Affect | End result variations | Low | Excessive | Group equity | Medium |
Counterfactual Equity | Causal relationships | Very Excessive | Medium | Causal | Low |
Equal Alternative | Classification errors | Medium | Medium | Group equity | Medium |
Demographic Parity | Output distribution | Low | Excessive | Group equity | Excessive |
R-BiasScore | Statistical correlation | Medium | Excessive | A number of | Medium |
The BiasScore analysis methodology balances complete protection and sensible usability. Whereas specialised metrics may excel in particular situations, the BiasScore in NLP supplies versatility for common functions. The BiasScore metric overview demonstrates benefits in interpretability in comparison with extra advanced approaches.
Conclusion
The BiasScore analysis methodology supplies an important framework for measuring and addressing bias in AI programs. By implementing BiasScore for bias detection, organizations can construct extra moral, honest, and inclusive applied sciences. The BiasScore within the NLP area continues to evolve, with new methods rising to seize more and more refined types of bias.
Transferring ahead, the Bias Rating analysis methodology will incorporate extra subtle approaches to intersectionality and context sensitivity. Standardization efforts will assist set up a constant BiasScore in NLP practices throughout the trade. By embracing these instruments at the moment, builders can keep forward of evolving expectations and construct AI that works pretty for everybody.
Steadily Requested Questions
BiasScore particularly measures prejudice or favoritism in mannequin associations or outputs. BiasScore in NLP sometimes examines embedded associations, whereas equity metrics may have a look at prediction parity throughout teams.
It’s best to apply the BiasScore for bias detection at a number of phases: throughout preliminary growth, after vital coaching updates, earlier than main releases, and periodically throughout manufacturing.
Sure, the BiasScore analysis methodology helps compliance with rising AI laws. Many frameworks require bias evaluation and mitigation, which BiasScore in NLP straight addresses.
For LLMs, template-based testing with the BiasScore works significantly nicely for bias detection. This entails creating equal prompts that adjust solely by protected attributes.
In case your mannequin exhibits regarding BiasScore in NLP, think about knowledge augmentation with counterfactual examples, balanced fine-tuning, adversarial debiasing methods, or post-processing corrections. The Bias Rating analysis methodology suggests focusing on particular bias dimensions relatively than making common modifications.
Login to proceed studying and revel in expert-curated content material.